|
 |
|
Fingerprint Technology Whitepaper |
|
SCOPE FingerTec technology is an advanced fingerprintmatching algorithm that ensures algorithm that ensures accuracy and security when used as an authentication method. FingerTec technology is the foundation for all authentication solutions from FingerTec and operates seamlessly with many third-party security applications, smart cards and biometric readers on the market. This article describes the principles and advantages of FingerTec technology. |
|
|
Using biometrics to verify identity means using a physical characteristic such as face,
voice or fingerprints to authenticate an individual’s claimed identity. Fingerprint matching is by far the most successful biometric technology because of its ease of use, nonintrusiveness and reliability. Fingerprints consist of ridges and valleys formed in complex patterns that are unique for every person and thereby provide an optimal verification method. This article discusses two main algorithm families commonly used to recognize fingerprints: minutia based and pattern based matching. These two methods evaluate fingerprint images in different ways; minutia matching compares specific details within the fingerprint ridges while pattern matching compares the overall characteristics of the fingerprints. As will be shown in this article, both methods have advantages and disadvantages. FingerTec continued research and development work has led to a more reliable and efficient fingerprint technology, the FingerTec solution.
FingerTec technology is designed for those integrated solution company, computer manufacturer, PDA manufacturer and other professional time and attendance, door access security, network security manufacturer that required ultimate fingerprint algorithm. FingerTec is a reliable technology where it has been well-research and developed for more than 15 years, near 10 millions of users has been using it.
FingerTec has tested and compared with almost all international fingerprint algorithm, and now it is compatible with most of the fingerprint sensor, with 360° rotation, high identification speed, and suitable for any public or defence application with a low expectation on fingerprint image (>=200dpi). A typical example is a national or sub-national ID card, where the template on the ID card will be matched against live fingerprint images from a broad variety of sensors. The FingerTec requires small amount of memory, where whole matching algorithm required just 350KB, allowing low-cost DSP or CPU-based hardware product is possible equipped with Biometric technology. |
|
|
|
Fingerprint Matching Methods |
Minutia Matching Every fingerprint consists of a number of ridges and valleys. Ridges are the upper skin layer segments of the finger and valleys are the lower segments. The ridges form socalled minutia points; ridge endings-where a ridge ends-and ridge bifurcations-where a ridge splits. At registration-enrollment-the minutia points are located (figure 1) and the relative positions to each other and their directions are recorded. This data forms the template, the information later used to authenticate a person. At the matching stage (figure 2), the incoming fingerprint image is pre-processed and the minutia points are extracted. The minutia points are compared with the registered template, trying to locate as many similar points as possible within a certain boundary. The result of the matching is usually the number of matching minutiae. A threshold is then applied, determining how large thisnumber needs to be for the fingerprint and the template to match. |
|
|
Figure 1: Enrolment of minutia points. |
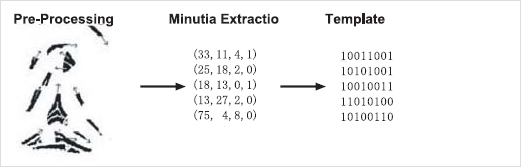 |
|
Figure 2: Verification using minutia points. |
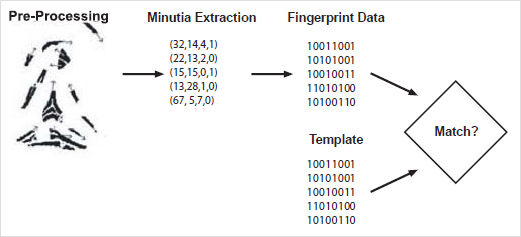 |
|
|
Pros: • Used in AFIS applications
• Well-known and well-researched method
• Algorithm is well suited for 1-many matching |
|
|
Cons:
• Cannot be used with all fingerprint sensor technologies, since it puts high demands on sensor resolution and sensor size. Gives poor results with fingerprint sensors less specified than AFIS grade.
• People with no or few minutia points (special skin conditions) cannot enroll or use the system. The number of minutia points can be a limiting factor for security of the algorithm.
• Can be confused by false minutia points (areas of obfuscation that appear due to low-quality enrollment, imaging, or fingerprint ridge detail). |
|
|
|
Pattern Matching
One intrinsic property of pattern matching algorithms is that overall fingerprint characteristics are taken into account, not only individual points. Fingerprint characteristics can then include sub-areas of certain interest including ridge thickness, curvature, or density. Due to this increased depth of data a pattern-based algorithm is less dependent on the size of the fingerprint sensor and is independent of the number of minutiae points in a fingerprint. Pattern-based algorithms do not, to the same extent as minutia-based methods, suffer from difficulties of recognizing a finger with varying fingerprint quality.
The graphical image is obtained from a capture device to distinguish it from a template stored in a database. Processing software examines the fingerprint image and locates the image center, which may be off-center from the fingerprint core. The image is then cropped a fixed distance around this graphical center. The rectangle in Figure 3 details this cropped region. The cropped region is then compressed and stored for subsequent match.
The verification procedure (figure 4) begins with the pre-processing of the incoming fingerprint image. The registered small images from the template are then compared with the fingerprint image to determine to what degree the template matches the image. A threshold describing the smallest allowable deviation is then used to decide if the finger matches the stored template. |
|
|
Figure 3: Enrolment with pattern-based algorithm |
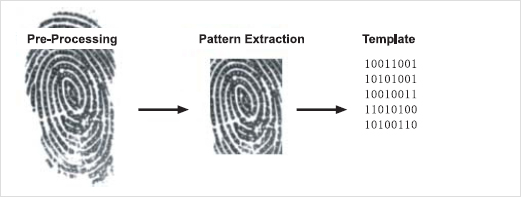 |
|
Figure 4: Verification using pattern-based algorithm |
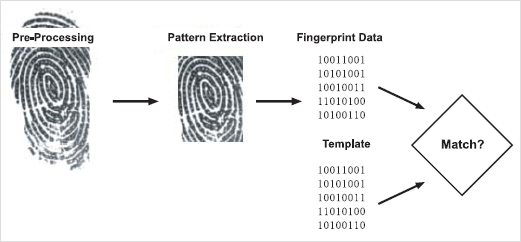 |
|
|
Pros:
• Works well with all known fingerprint sensor types
• All fingerprints possible to capture can be enrolled, even those with no or very few minutia points
• Well suited for implementations with scarce computing resources e.g. a smart card. |
|
|
Cons:
• Cannot make use of existing AFIS databases (can use raw images though)
• Not optimized for identification (1 to many searches in a database)
• Can be confused by false minutia points (areas of obfuscation that appear due to low-quality
enrollment, imaging, or fingerprint ridge detail). |
|
|
|
Minutia vs Pattern Matching |
|
Minutia |
Patterm |
Definition |
Analyzes the points at which the ridges on the fingerprints split, intersect or end. |
Graphical comparison of fingerprint image. |
How it works |
Capture device analyzes the fingerprint image to determine the location of the fingerprint core, the pattern type (i.e., right loop, let arch, etc.), estimates the quality of the ridgelines and extracts the point in which the ridges split, intersect or end. These points are called minutia. |
Graphical center of fingerprint image (not necessarily defined by the fingerprint core) is cropped a fixed distance and compressed for subsequent match. The greater the difference between the stored template and the live comparison, the less likely the match. |
Template size |
Small template size (can be as small as 120 bytes; average size is 350 bytes). Template size can be controlled by specifying the number of minutia to be analyzed. |
Relatively large template size (500-700 bytes when compressed). Cannot esily control template size without Compromising accuracy. |
Search speed |
Directly related to template size; the smaller the template, the faster
the search speed. |
Sensitivity to Physical Changes |
Less sensitive due to the fact that only 30% of the available minutia are required for matching. Cuts and acars usually will not affect all the minutia on the fingerprint. |
If the acar or blemish affects the region ot the fingerprint image that was scarred, a new template may be required. |
Template Efficacy |
Can extract minutia from partial prints (as often found in crime scenes), making it more feasible to criminal related applications. |
Requires the same central region to be patterned for the match to occur. Not suitable for criminal applications where partial prints are often used as the basis for investigation. |
Sensitivity to time |
Less sencitive to changes over time. |
More sensitive to physical changes and differences in the fingerprint placement on the sensor-both which become greater over time. |
Standard |
X.509 AAMVA B10.8 |
None |
Leading Vendors |
Identix, FingerTec |
BioScrypt, Precise Biometrics, Digital Persona, Sony |
|
|
Fingertec Matching Algorithm |
Minutiae matching technique is used in FingerTec technology. The algorithm takes advantages of the minutia points. This makes FingerTec very efficient at dealing with large database, even fingerprints of low quality. |
|
|
Benefits of the FingerTec matching algorithm: |
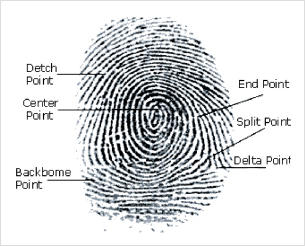 |
|
|
|
1. |
When using FingerTec for 1 - N identification (2000-6000 fingerprints), there is no requirement to enter a name or a PIN number. Identification can be carried with ease within 1-5 seconds (Minimum hardware requirement of Pentium III, 900MHz PC). |
|
|
2. |
FingerTec allows easy integration to end user applications. Through various image files, it can support many fingerprint sensor types (with resolution of >= 300DPI). |
|
|
3. |
FingerTec algorithm is able to filter off noises, ridge ruptures and stuck ridges from fingerprints. Even for fingerprints that are with bad quality (dirty, cuts, scars, dry, wet or damaged), the algorithm is able to extract accurate minutiae points. |
|
|
4. |
FingerTec Matching algorithm allows of 360° rotation of live capture for identification or verification. With state-of-the-art techniques, the match can still be conducted at high speed despite the different orientation angles of the finger (Approximate Matching Speed = 3000 match/sec) even for fingers with low minutiae count (<=10), a fingerprint normally would have >= 15 minutae counts. |
|
|
5. |
FingerTec matching algorithm does not require complete minutiae points such as (core, delta, etc), it can successfully identify based on several essential or critical minutiae points. |
|
|
6. |
FingerTec divides fingerprints into 5 categories (eg: Arch, left loop, right loop, tented arch, whorl), when categorization is used, the matching speed will be greatly increased. |
|
|
7. |
FingerTec matching algorithm source code is simple, only 350Kbytes of program space is required, therefore it is easily fit into any PC hardware and software programs. |
|
|
Algorithm Performance |
FingerTec has been gone through many testing using 4 types of sensor (YLC, DFR200, U.ARE.U, Authentec) where 2000 fingerprints are obtained to test the performance of the FingerTec matching algorithm. Each sensor is tested with 500 fingerprints, each of them is then cross match with the other of the 2000 fingerprints, around 4,000,000 matches were perform, and obtained the following results:
Fingerprint template size : 256 or 1024 bytes
Rotation : 0 - 360 degrees
FAR : <= 0.001%
FRR : <= 2.0%
Enrollment time : 0.5 seconds
Matching Speed : 2000-4500 matches/sec
Livescan Image : > = 300DPI
Statistical measures such as the false acceptance rate (FAR, also known as False Match Rate), and the false rejection rate (FRR, also known as False Non-Match Rate) are often cited in order to quantify the “classification strength” of the biometric algorithm. However, it is very important not to confuse the FAR measure with the level of security provided by a biometric verification system. A system is never more secure than its weakest link and fingerprint verification systems in general have matured far beyond the biometric algorithm being a weak link. FAR and FRR are diametrically opposed, increasing FAR will lower FRR and vice-versa.
For any biometric system, user training will in general have a positive impact on the FRR and failure to enroll (FTE) rate, as captured biometric data will present high variability. Therefore, it is necessary to train user-knowledge and skills to reach optimum performance.
In particular, care must be taken to optimise the enrolment process to get the best possible fingerprint template by user feedback and advanced image processing to determine fingerprint quality. The enrollment process is by far the most important step in the usage of a biometric recognition system. This is because the biometric template, which is the result of the enrolment process, is what the system will use to compare against all subsequent live fingerprint samples.
Using a database for determining FRR is however not obviously a method that in all situations correlates to field usage of the system. One of the reasons for this is that fingerprints in a database are static, so for instance user feedback cannot be simulated. FingerTec continuously runs field tests of the complete system to get statistics for continued improvement of the matching performance. |
|
|
|